Data centric AI vs Model centric AI
Explore the dynamic world of artificial intelligence with a focus on Data-Centric AI and Model-Centric AI, each offering unique advantages and challenges. Discover how the blend of these approaches is shaping the future of AI for the benefit of humanity.
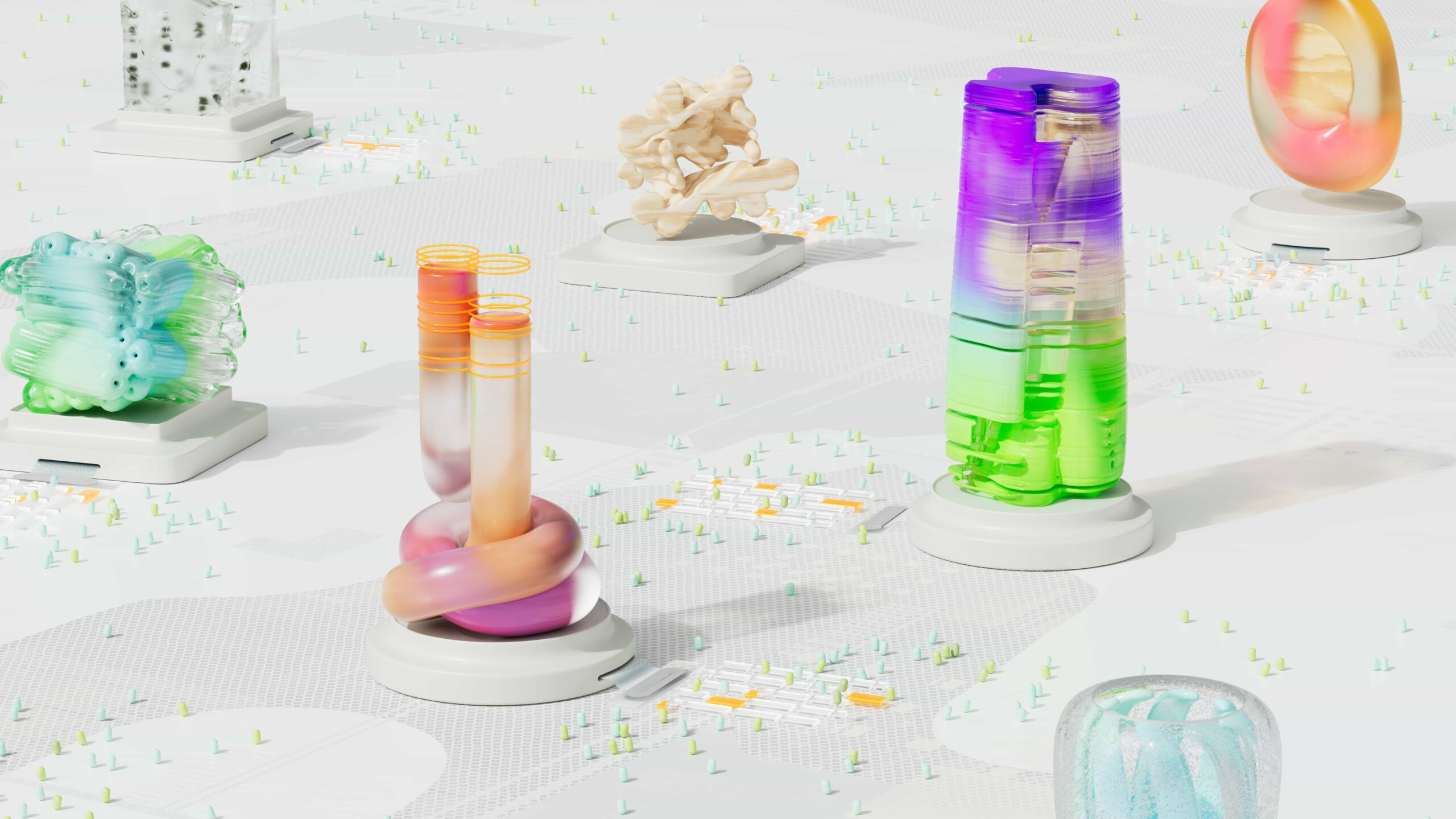
Introduction
In the rapidly evolving world of artificial intelligence (AI), two prominent approaches have emerged: Data-Centric AI and Model-Centric AI. These paradigms dictate how AI systems are developed, deployed, and optimized, each offering distinct advantages and challenges. By delving deep into these approaches, we can gain insights into the future trajectory of AI, ensuring that the technology evolves in ways that are both powerful and beneficial to humanity.
Data-Centric AI
Data-Centric AI places a strong emphasis on the quality, quantity, diversity, and management of data. In this approach, the focus is on curating vast, high-quality datasets, pre-processing, and cleaning data, ensuring data privacy, and enhancing the overall data pipeline. It operates on the principle that superior data leads to superior AI performance, even with simpler models. This paradigm has become increasingly popular due to its capacity to address complex, real-world challenges efficiently.
The Significance of Data: Data is considered the lifeblood of AI systems. It is used to train, validate, and test models, playing a crucial role in determining their accuracy and efficiency. In the data-centric view, efforts are concentrated on enhancing data annotation, collection, and processing. Various tools and methodologies, such as data augmentation, synthetic data generation, and differential privacy, are employed to increase the dataset’s size and quality.
Advantages of Data-Centric AI:
- Enhanced Performance: With high-quality data, AI models can achieve better accuracy and generalization, reducing overfitting.
- Flexibility: This approach allows for adaptability, as updating and improving the dataset can lead to improved AI performance without changing the underlying model.
- Privacy Preservation: With a focus on data, methods like federated learning can be employed to train models on decentralized data, ensuring privacy.
Model-Centric AI
In contrast, Model-Centric AI focuses on the development of complex, highly efficient models. This approach is rooted in the belief that advancements in AI are driven primarily by more sophisticated algorithms and architectures. Model-Centric AI has given rise to models like GPT-3 and BERT, characterized by their vast number of parameters and exceptional performance across various tasks.
The Algorithm’s Reign: In the Model-Centric approach, algorithm development is paramount. It revolves around the innovation of new architectures, optimization techniques, and training methodologies. These complex models often require extensive computational resources and are trained on massive datasets, albeit sometimes with less emphasis on data quality and diversity.
Advantages of Model-Centric AI:
- State-of-the-Art Performance: Complex models often achieve benchmark-setting results across numerous AI tasks.
- Transfer Learning: These models can be fine-tuned on smaller, task-specific datasets, reducing the need for extensive data collection.
- Robustness: With the right architecture, model-centric AI can be resilient to noise and variations in data.
The Intersection: The dichotomy between Data-Centric and Model-Centric AI isn’t rigid. In practice, a synergy between quality data and powerful models is often the key to achieving optimal AI performance. Combining the strengths of both paradigms can lead to AI systems that are accurate, efficient, and capable of tackling a diverse array of challenges.

Examples: Numerous real-world applications illustrate the benefits of a balanced approach. In healthcare, Data-Centric AI ensures the privacy and quality of patient data, while Model-Centric AI drives the development of models capable of complex tasks like diagnostic imaging. In autonomous vehicles, quality data ensures safety and reliability, while sophisticated models navigate the complexities of real-time decision-making.
Future Trends: As AI continues to evolve, a holistic approach that embraces both data and model-centric perspectives is likely to prevail. The advent of models like OpenAI’s GPT-3, with its 175 billion parameters, showcases the power of sophisticated architectures. However, the parallel emphasis on data quality, privacy, and management underscores the critical role of data.
Conclusion:
Navigating the future of AI requires a nuanced understanding of the Data-Centric and Model-Centric approaches. Each brings distinct strengths to the table, driving AI’s advancement in unique ways. By harmonizing these paradigms, we can pave the way for AI systems that are not only powerful and intelligent but also ethical, equitable, and beneficial to all of humanity. The blend of these approaches promises a future where AI’s potential is fully realized, ushering an era where technology and human welfare coalesce seamlessly.
In this evolving landscape, the role of AI practitioners, policymakers, and ethicists becomes paramount. They are the custodians of a future where AI is molded to be a robust tool, a companion that augments human capabilities, and a guardian that ensures fairness, equity, and justice in every application. The dance between data and models is intricate but mastering their harmony will determine the melody of AI’s future symphony.
You might be interested in diving deeper into the world of artificial intelligence by exploring the differences between Data-Centric AI and Model-Centric AI. Speaking of data-centric approaches, you might be interested in learning more about the importance of data quality and management by checking out the article on data quality. On the other hand, if you want to understand the significance of algorithm development in Model-Centric AI, you can read up on various optimization techniques and training methodologies by visiting the page on algorithm development. By delving into these topics, you can gain a deeper understanding of how AI systems are evolving and the potential impact they can have on society.