OpenAI’s o3 Model: The Next Leap Toward AGI or Overhyped Increment?
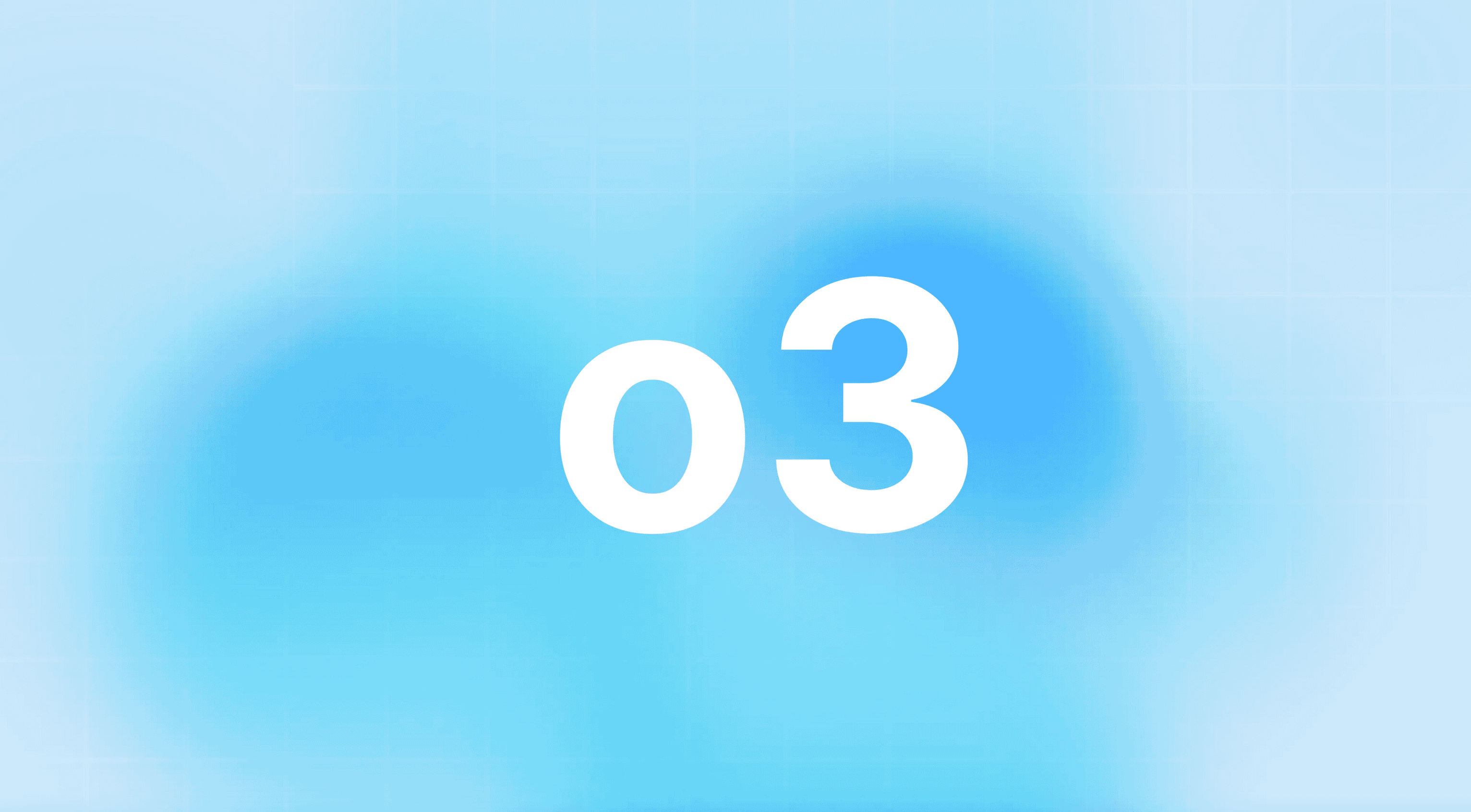
The unveiling of OpenAI’s o3 model has ignited discussions across AI circles, media outlets, and social networks about its implications for artificial general intelligence (AGI) and the broader trajectory of machine learning. As a model that achieved an unprecedented 87.5% on the ARC-AGI Semi-Private Evaluation—a benchmark historically hostile to AI systems—o3 represents a significant leap forward in capability. Yet, its debut has also raised important questions about the state of AGI research, the role of benchmarks, and the practical and ethical implications of advancing AI technologies.
In this article, we’ll unpack the technical achievements of o3, assess the broader implications of its advancements, and explore the ongoing debates around AGI’s feasibility and impact.
Breaking Down the o3 Achievement
The o3 model stands out not only for its superior performance on the ARC-AGI benchmark but also for its success in solving challenges across a range of STEM domains.
1. A New Benchmark in Problem-Solving
The ARC-AGI benchmark, designed to evaluate a system’s ability to handle novel, abstract tasks, has long been a litmus test for progress toward AGI. Historically, GPT models struggled with this test, scoring near-zero or in the single digits. For instance:
- GPT-3 (2020): 0%
- GPT-4o (early 2024): 5%
- o1-preview (2024): 18%
o3’s 75–88% range is a dramatic leap that dwarfs prior iterations and even challenges the performance of human experts in certain domains.
2. Outperforming Human Specialists
According to OpenAI, o3 excelled in “Google-proof” doctoral-level science questions, achieving 87.7% accuracy, which surpasses the typical performance of human Ph.D. experts. Its success extends beyond isolated benchmarks; the model also solved 25% of questions in the Frontier Math benchmark, a task other competitive models barely scratched.
These achievements paint a picture of an AI system with growing competence in tasks previously thought exclusive to humans. However, critics and skeptics urge caution in interpreting these results as definitive indicators of AGI.
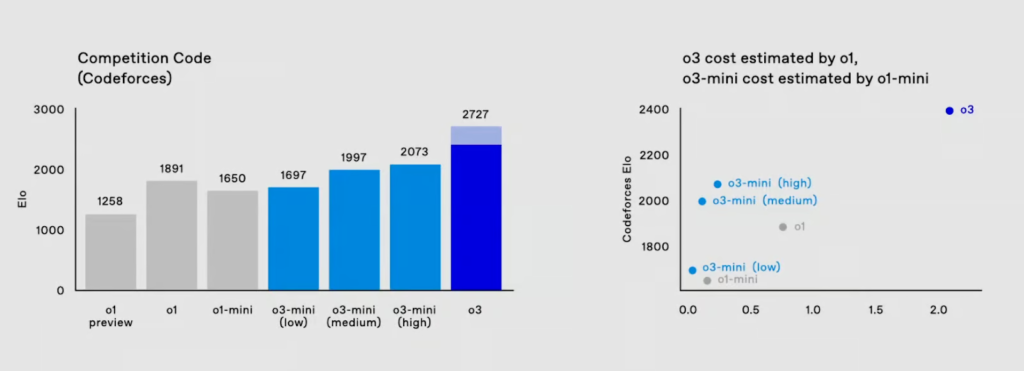
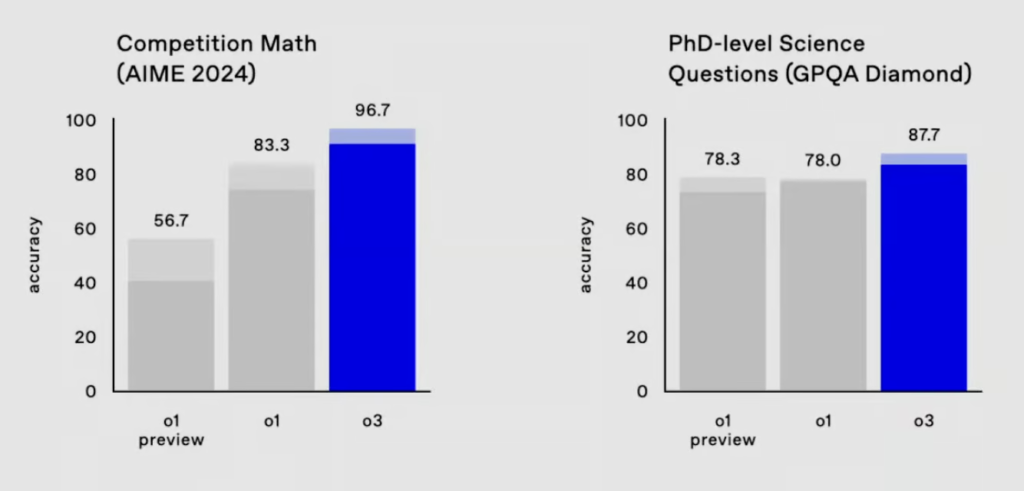
What Does This Mean for AGI?
AGI remains an elusive and contentious goal in AI research. Definitions vary, but at its core, AGI refers to autonomous systems capable of outperforming humans in most economically valuable tasks. While o3’s performance is remarkable, the ARC Prize organization itself notes that “passing ARC-AGI does not equate to achieving AGI.” This distinction underscores the complexity of the debate surrounding o3.
1. Benchmark Mastery vs. True Intelligence
o3’s success on ARC-AGI raises an important philosophical question: Does excelling at benchmarks equate to approaching human-like intelligence? The skepticism stems from the fact that models trained on specific datasets—no matter how sophisticated—may still struggle with generalization and intuitive reasoning, hallmarks of human intelligence.
- Proponents argue that training on large, complex datasets is analogous to human learning through practice and exposure. In this view, o3’s mastery of benchmarks reflects meaningful progress.
- Skeptics, like cognitive scientist Gary Marcus, caution that reliance on publicly available training sets like ARC-AGI-1 may inflate perceived progress. Marcus calls for rigorous external review to validate claims and assess the robustness of o3’s capabilities beyond benchmarks.
2. The Limits of Current AI Systems
Despite its impressive results, o3 reportedly still fails on some “very easy tasks,” highlighting fundamental differences between machine and human cognition. This discrepancy suggests that while models like o3 can excel in narrowly defined tasks, they may lack the adaptability and creativity required for true AGI.
Implications for STEM, Jobs, and Society
The o3 model’s success has sparked anxiety among professionals, particularly in STEM fields like coding and mathematics, where the system already rivals competitive human experts.
1. Coding and STEM Workflows
As o3 demonstrates proficiency in advanced programming tasks and mathematical problem-solving, its impact on these domains could be transformative:
- Automation of Routine Coding Tasks: Developers may increasingly rely on models like o3 to handle boilerplate code or troubleshoot complex bugs.
- Augmenting Scientific Research: o3’s ability to tackle doctoral-level problems suggests potential applications in academia and industry, where AI could accelerate discovery in fields like drug development, engineering, and physics.
2. Job Displacement Concerns
The flip side of automation is the potential for displacement:
- Coders and other STEM professionals worry that as AI systems become more competent, their own expertise may be devalued.
- OpenAI and other stakeholders must address these concerns through proactive policies, such as upskilling programs, to ensure a balanced transition to an AI-enhanced workforce.
3. Broader Ethical and Societal Considerations
Beyond economic implications, the advancement of models like o3 raises ethical questions:
- Bias and Fairness: Will AI systems amplify existing biases in STEM fields, or will they help to mitigate them?
- Accountability in Decision-Making: As AI systems increasingly contribute to high-stakes fields like medicine or law, ensuring transparency and accountability becomes critical.
The Debate Over Progress in Generative AI
o3’s debut also challenges the prevailing narrative that generative AI (genAI) had hit a plateau. Recent critiques suggested diminishing returns in performance improvements, with advancements becoming more incremental and less impactful.
- Renewed Optimism: o3’s achievements suggest that significant leaps in performance are still possible, even if they come with rising costs and complexity.
- Caveats About Scalability: As models grow larger and more powerful, they also become more expensive to train and deploy. This raises questions about the sustainability of the current trajectory in AI research.
A Rebuttal to the Skeptics
Some observers argue that the skepticism surrounding o3 overlooks its broader potential:
- Transformational Capabilities: Even if o3 falls short of AGI, its ability to tackle challenging STEM tasks could unlock breakthroughs in science, engineering, and beyond.
- A Roadmap for AGI: Each leap in capability provides valuable insights that inform future research, making AGI more achievable in the long run.
Where Do We Go From Here?
The debut of OpenAI’s o3 marks a pivotal moment in the AI landscape, with implications that extend far beyond technical benchmarks. Whether it represents a meaningful step toward AGI or simply another milestone in narrow AI development, the conversation it has sparked is a critical one.
1. Responsible Development and Deployment
To maximize the benefits of systems like o3 while minimizing risks, stakeholders must prioritize:
- Transparency in research and reporting
- External validation of results
- Ethical frameworks for deployment
2. Collaboration Across Sectors
Advancing AI responsibly will require input from academia, industry, and government to ensure that progress aligns with societal needs and values.
3. Bridging Hype and Reality
Finally, researchers, media, and the public must approach developments like o3 with a balanced perspective, celebrating achievements without succumbing to uncritical hype.
Takeaways
OpenAI’s o3 model is undoubtedly a landmark in AI research, pushing the boundaries of what generative models can achieve. Yet, it also serves as a reminder of the challenges that lie ahead in the quest for AGI. As we navigate this evolving landscape, we must remain vigilant, thoughtful, and collaborative in shaping the future of AI.