Understanding Chain of Thought (CoT)
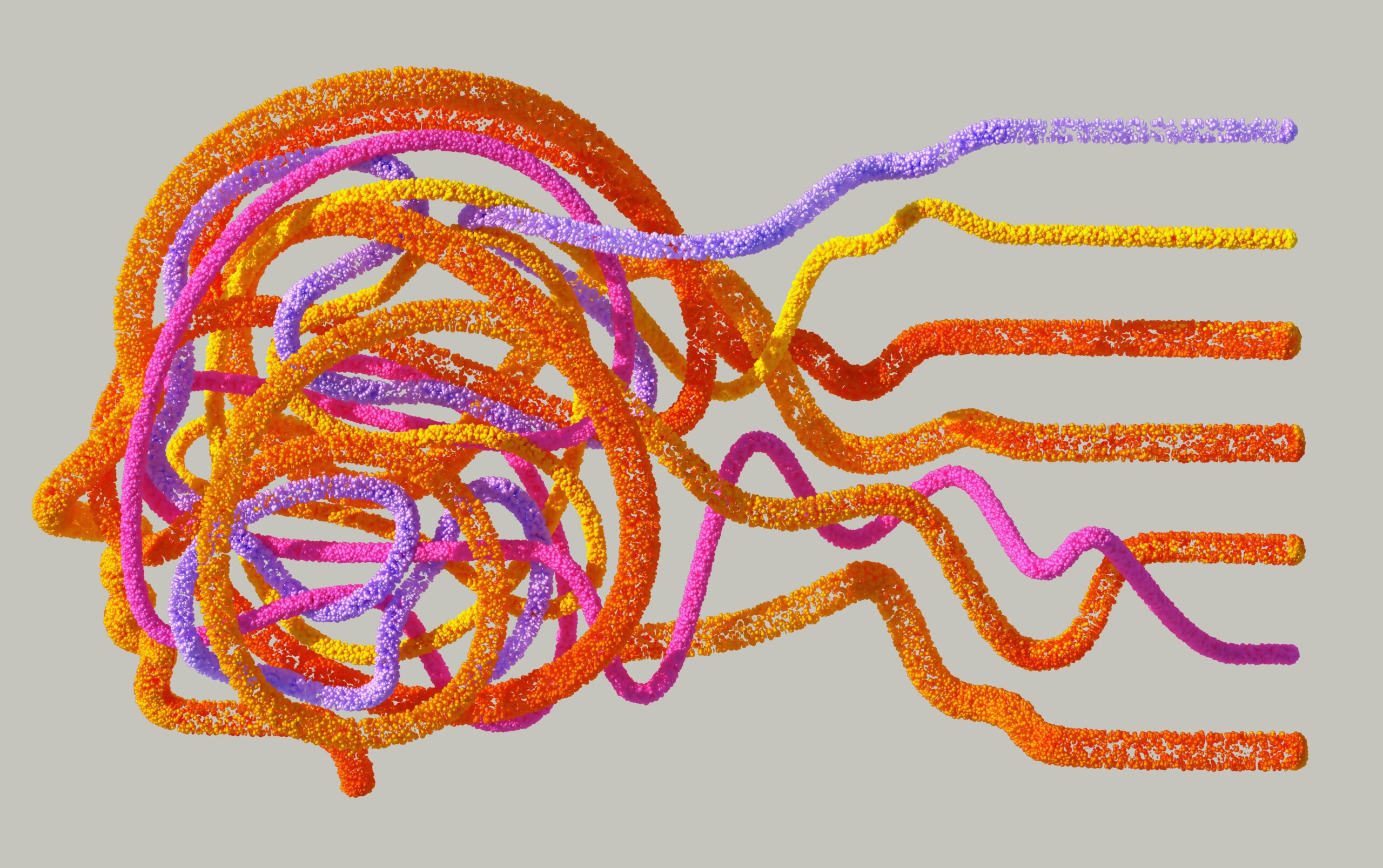
- The Foundation of Chain of Thought Prompting: Human-like Reasoning in AI
- Key Variants of Chain of Thought Prompting
- Advantages of Chain of Thought Prompting in AI
- Challenges and Limitations of Chain of Thought Prompting
- Practical Applications of Chain of Thought Prompting
- Future of Chain of Thought Prompting in AI Development
In the realm of artificial intelligence (AI) and large language models (LLMs), Chain of Thought (CoT) prompting has emerged as a revolutionary approach, mirroring aspects of human reasoning. This technique allows AI models to break down complex problems into systematic, logical steps, resembling the cognitive processes humans naturally employ in problem-solving. Unlike straightforward or basic prompts, which typically focus on generating direct answers, CoT prompting requires the AI to construct coherent reasoning chains, weaving through intermediary steps that lead to an insightful and accurate conclusion. This nuanced approach is not only transforming AI’s problem-solving capabilities but also opening up vast potential in applications from customer service to scientific research.
As we delve deeper into the architecture and applications of CoT, we also confront its limitations and challenges. However, advancements in this domain signal an exciting path forward, with continuous refinement allowing AI to handle increasingly complex reasoning tasks in a transparent and interpretable manner. Let’s explore the principles, types, benefits, limitations, and promising use cases of CoT to understand how this prompting method is shaping the future of artificial intelligence.
The Foundation of Chain of Thought Prompting: Human-like Reasoning in AI
At its core, Chain of Thought prompting draws on the cognitive strategy of decomposing a problem into manageable, sequential steps. This enables an AI model not only to generate a response but to explain how it arrived at that conclusion. Consider the example of asking an AI, “Why is the sky blue?” A simple prompt might elicit the direct answer, “Because of Rayleigh scattering.” However, a CoT prompt leads the AI to first explain Rayleigh scattering, why shorter wavelengths scatter more effectively, and how this affects our perception of color. This stepwise reasoning allows the model to mirror human analytical processes, making the response both richer and more informative.
The utility of CoT is evident in its ability to handle nuanced queries. By breaking down complex tasks into intermediate steps, CoT prompting effectively tackles multi-step reasoning challenges. For instance, solving a mathematical problem or answering a multi-part question becomes a structured process, with each intermediate answer building toward the final solution.
Key Variants of Chain of Thought Prompting
The evolution of CoT has given rise to several variants, each addressing distinct challenges and enhancing the capabilities of large language models:
- Zero-shot Chain of Thought: In zero-shot CoT, the AI model addresses problems it hasn’t explicitly trained for, leveraging its embedded knowledge to deduce logical steps. For example, in identifying the capital of a country bordering France with a red and white flag, a zero-shot CoT-enabled model could conclude that the answer is Switzerland by analyzing geographical and visual knowledge.
- Automatic Chain of Thought (Auto-CoT): This variant automates the generation of reasoning paths, reducing the manual input needed to guide the model. Auto-CoT is particularly advantageous for scaling AI applications, making the CoT method accessible across diverse tasks by generating intermediary steps without human intervention.
- Multimodal Chain of Thought: By incorporating various input modalities (e.g., text, images), multimodal CoT allows AI models to synthesize information across different formats. For example, an AI model analyzing an image of a beach and responding to a question about its popularity could integrate visual details with its understanding of seasonal trends to form a comprehensive answer.
These variants collectively enhance CoT’s adaptability and potential, enabling AI to tackle a broader range of tasks while deepening its interpretative abilities.
Advantages of Chain of Thought Prompting in AI
The benefits of CoT prompting extend across a spectrum of applications, from improving output accuracy to enhancing interpretability:
- Enhanced Accuracy in Complex Tasks: CoT’s systematic approach improves the precision of AI responses, particularly in tasks requiring layered or sequential reasoning. The structure of CoT helps the model break down convoluted tasks, increasing the reliability of the final output.
- Transparency and Interpretability: The intermediate reasoning steps generated through CoT make the model’s decision-making process more transparent. For users, this is particularly useful, as it allows them to follow the logical path taken by the model, which can be invaluable in fields like medicine or law where traceable reasoning is essential.
- Multi-Step Reasoning Capability: By working through each component of a problem in sequence, CoT enables a more detailed response. This approach is highly valuable for applications in education, where understanding the process is crucial to learning.
- Broad Applicability: CoT’s versatility makes it suitable for a wide range of tasks, from logical reasoning to creative problem-solving. Its ability to handle everything from arithmetic tasks to abstract questions highlights its flexibility and utility.
- Attention to Detail: Similar to stepwise teaching methods, CoT prompting promotes meticulous breakdowns, making it particularly useful in educational and scientific contexts where detailed understanding is essential.
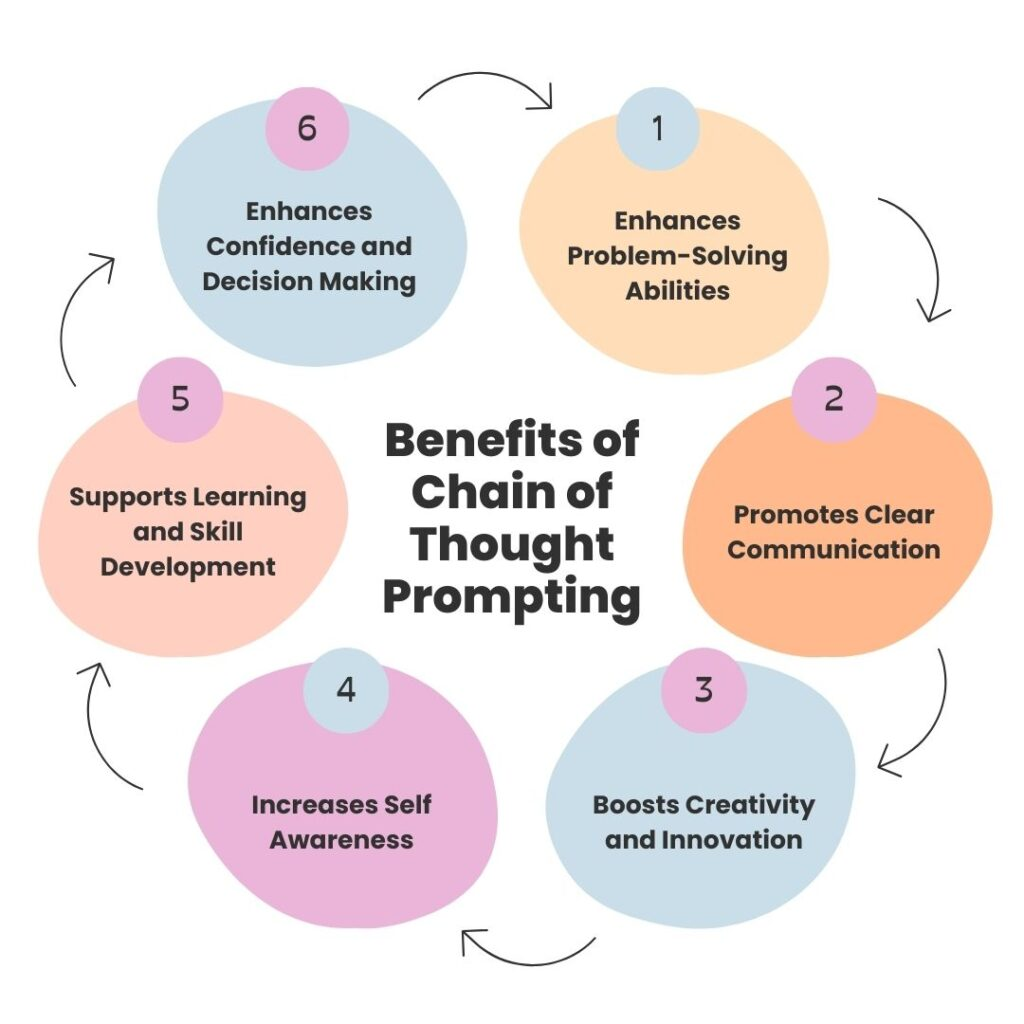
Source: Link
Challenges and Limitations of Chain of Thought Prompting
Despite its numerous advantages, CoT prompting is not without limitations:
- High-Quality Prompt Requirements: The effectiveness of CoT depends significantly on the quality of the initial prompts. Crafting these prompts often requires in-depth expertise, making CoT more labor-intensive than simpler prompt strategies.
- Increased Computational Demands: The generation of reasoning steps entails a greater computational cost, as each step requires processing power. This can increase both the time and financial cost of using CoT, particularly for complex, high-stakes applications.
- Risk of Misleading Reasoning Paths: An inherent risk in CoT is the potential for plausible-sounding but incorrect reasoning paths, where the AI may generate intermediate steps that sound logical but lead to erroneous conclusions.
- Labor and Complexity in Prompt Design: Designing effective CoT prompts is often challenging and labor-intensive, requiring detailed domain knowledge. This can make CoT less accessible for some applications or for those without deep technical expertise.
- Potential Overfitting: There is a risk that AI models may overfit to specific styles or patterns in the prompts, limiting their generalizability across varied tasks. This reduces CoT’s flexibility and can affect performance in unpredictable scenarios.
- Difficulty in Evaluating Reasoning Quality: Assessing the quality of reasoning steps generated by CoT models can be challenging, given the subjective nature of human reasoning. Although intermediate steps can enhance interpretability, qualitative improvements in reasoning are difficult to measure objectively.
Practical Applications of Chain of Thought Prompting
The versatile utility of CoT prompting is reflected in its applicability across numerous domains, offering transformative potential in industries ranging from customer service to research and education.
- Customer Service Chatbots: Advanced AI chatbots using CoT can break down customer queries into understandable steps, offering more accurate and context-sensitive responses. This structured approach reduces dependency on human intervention, potentially improving both user satisfaction and service efficiency.
- Scientific Research and Innovation: CoT supports researchers by organizing complex thought processes, aiding in the formulation of hypotheses and streamlining the discovery process. In research-intensive fields, CoT could accelerate innovation by helping scientists to systematically address multifaceted problems.
- Content Creation and Summarization: CoT aids in producing coherent, logically structured content by organizing information and generating structured outlines. This is particularly valuable in summarization tasks, where logical flow is essential to create concise and informative summaries.
- Educational Support: In educational technology, CoT can guide students through step-by-step explanations, reinforcing learning by focusing on the reasoning process as much as the answer. This is especially valuable in subjects like mathematics, where process understanding is as crucial as arriving at the correct answer.
- AI Ethics and Decision-Making: In applications where ethical considerations are essential, CoT provides a transparent reasoning process, allowing for scrutiny and alignment with ethical standards. This transparency ensures that AI-driven decisions can be verified and held to ethical benchmarks, which is critical in fields like healthcare and finance.
Future of Chain of Thought Prompting in AI Development
Chain of Thought prompting represents a groundbreaking step in advancing AI’s reasoning and problem-solving capabilities. By emulating human cognitive processes, CoT opens the door to more nuanced, transparent, and interpretive AI models that can tackle a wide array of complex challenges. With continued innovation, we can expect CoT’s variants to further refine AI’s reasoning abilities, addressing current limitations and expanding its applications across new and diverse fields.
As CoT evolves, we anticipate that its integration into AI frameworks will enhance the decision-making processes in crucial areas, promoting safer, more accountable, and more ethically aligned AI. This methodology offers the promise of enabling AI systems to understand and respond to complex real-world challenges, transforming how we approach problem-solving in the digital age.